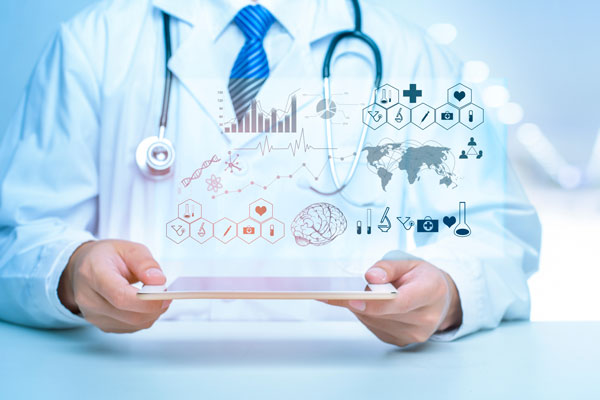
Healthcare Analytics:
How to Tap into the Data that Will Drive Meaningful Change
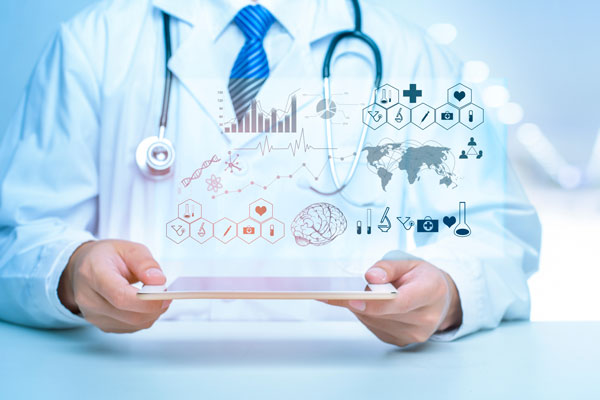
Healthcare Analytics: How to Tap into the Data that Will Drive Meaningful Change
Introduction
Nearly every healthcare system has some form of analytics in place. But as they have found, it’s one thing to collect data, and wholly another to make actionable use of it. That’s the hard part.
For healthcare organizations, what’s the next step when it comes to analytics? Where do they go from here to gain true insights that move the needle in improving patient outcomes, reducing costs, and improving the experience of patients and clinicians?
Although healthcare systems have data feeds from various sources that can be mined for valuable insights, much of this data remains untapped. Unfortunately, despite their awareness of how valuable data-driven decision-making is, many organizations either lack the solutions necessary to capitalize on it, or simply just aren’t sure HOW to capitalize on it. In many cases, the amount of data has become overwhelming, and organizations aren’t sure which fraction of that data they need to focus on to get the results they desire.
To truly maximize ROI, hospitals need to mobilize their data at every level of their organizations. This means combining a comprehensive data solution that can handle system-wide needs with a company culture that prioritizes data democracy. Furthermore, healthcare systems need to take a step back and identify where their greatest weaknesses lie, and they need to work with experts who understand exactly how to use data to address those weaknesses.
In this white paper, we will discuss some of the ways healthcare systems can apply data analytics to not just amass data as many have done, but to take the rarer step of transforming operations and making meaningful improvements.
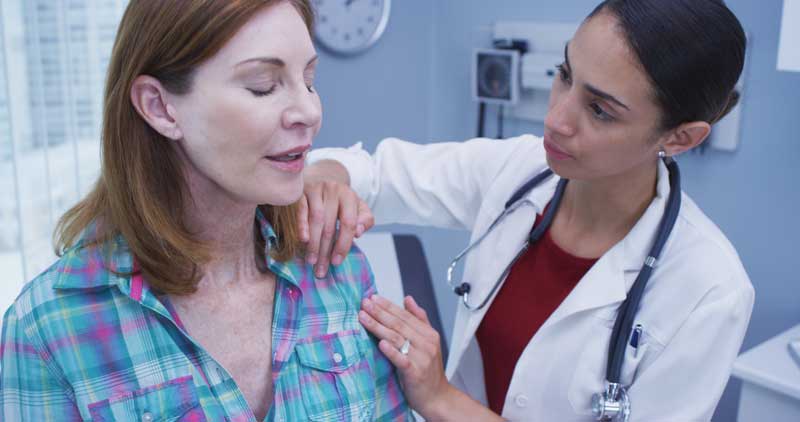
Patient leakage
On top of costing hospitals potentially millions in lost revenue, patient leakage—or when a patient receives care outside the hospital network—can also lead to delays in patient diagnosis or care, diminished visibility into the patient’s healthcare journey, and reduced care continuity.
In order to implement new strategies and address potential weaknesses, hospitals need to be able to identify what exactly is working in your network, and what isn’t. For some systems, a particular specialist may attract a significant number of referrals due to their reputation or niche. For others, a high volume of inbound referrals could be the result of strong existing relationships with other healthcare institutions or specific providers. Regardless of what’s bringing in your referrals, your data can reveal why it works and what you can do to capitalize on its success.
There are a few strategic measures your organization can start with to get a hold of your patient leakage challenges:
Network integrity: This is what percent of unique patient volume stays within your physician network. This functions as your overall patient leakage metric.
Patients you’ve lost to specialists from competitor care units: This can be displayed as percent of unique patient volume that receives future services from competitor facilities.
Individual physician capture rate: You could also measure percent of unique patient volume within your health system for each physician that interacts with your facility. This allows your administrators to initiate discussions with individual physicians who may be underutilizing your available facilities and services. The follow-up would then be to understand what these barriers to utilization are and how to overcome them.
Moving beyond physician-related metrics, providers can also utilize predictive analytics to identify patients who are likely to travel out-of-network for their treatment. For example, hospitals can review patterns of utilization for specialist consultations outside their network to formulate a plan with their own specialists for better patient engagement and retention. Likewise, demographic information regarding your patient population can reveal potential weaknesses within your provider network.
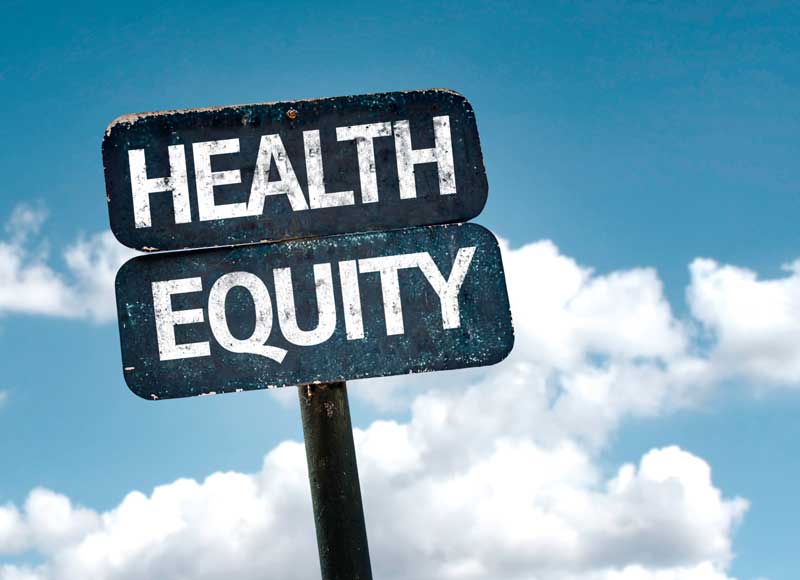
Health equity
The onset of the pandemic brought the topic of social determinants of health to the forefront as hospitals dealt with disproportionate caseloads amongst underserved communities. However, health inequity has plagued the healthcare industry for quite some time now. Black, Latinx/Hispanic, and American Indian and Alaska Native (AIAN) populations are less likely to have health insurance, more likely to face cost-related barriers to getting care, and more likely to incur medical debt.¹ Likewise, they are also more likely to die during or after pregnancy, suffer serious pregnancy-related complications, and more likely to lose children in infancy.²
Moving forward, improving population health and delivering care across the general public requires a nuanced understanding of each community and their individual challenges. The key to understanding these nuances is hidden in the data.
Data analytics can be employed to identify systematic opportunities for improvement across different demographics based on various quality-of-life indicators. Most of the biggest contributing factors are related to social perception, economic stability, and lifestyle, such as:
Subject matter being perceived as shameful
Poor prior experiences (whether locally or from location of origin)
Discomfort with different sex/race/language providers
Unfamiliarity with screening practices and general lack of education
Basic needs taking priority (e.g. prioritizing food and rent over doctor’s visits)
Inadequate insurance coverage
The easiest way to identify health inequity within your network is to measure demographic characteristics against medical outcomes of interest. Once you’ve identified demographic trends in your data related to specific outcomes (e.g. different races may experience different rates of pregnancy-related complications), hospitals can implement new strategies to address these disparities. While some of this demographic data is readily available (such as race and sex), other traits like education and income will likely require third-party sources such as state or local public health departments.
Furthermore, demographic information related to your patient volume can also provide insight into where your hospital should be directing additional resources to help reach underserved populations.
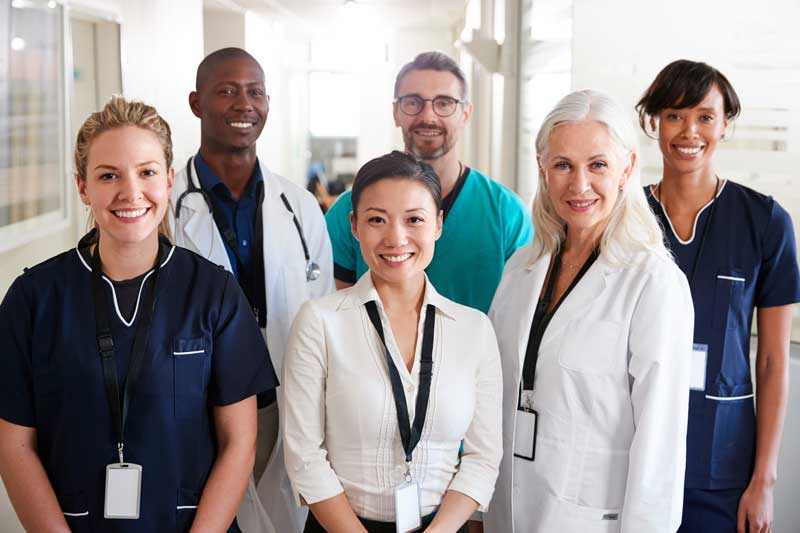
Staffing
Major disruptions like the COVID-19 pandemic have resulted in a substantial labor shortage in the United States—an event now referred to as The Great Resignation. As many as two in five nurses and one in four physicians intend to quit in the next two years,³ and the demand for healthcare workers is anticipated to grow by 45% between 2020 and 2030.⁴ Due to the expensive costs associated with recruiting and onboarding new employees, more and more healthcare systems are turning to HR data analytics for retaining their workforce.
With analytics, hospitals can measure their turnover and retention rates, gaining visibility into how many employees have left their company over a set period of time. These numbers can then be filtered down by whether or not the turnover was voluntary, how well-performing those employees were, and how turnover rates compare between different departments and managers. Understanding the context in which an employee left is crucial to addressing internal influences and reducing future resignations.
Additionally, in order to evaluate the risk of future resignations, hospitals can measure employee satisfaction and intent to stay through surveys, as well as evaluate compensation to ensure that employees aren’t leaving due to inadequate pay. This information can then be used to implement new policies and workflows or introduce incentives for employee retention.
With all that said, resignations are inevitable, and hospitals should be tracking metrics related to current openings and weaknesses in their workflow to identify where they need to direct their recruiting resources.
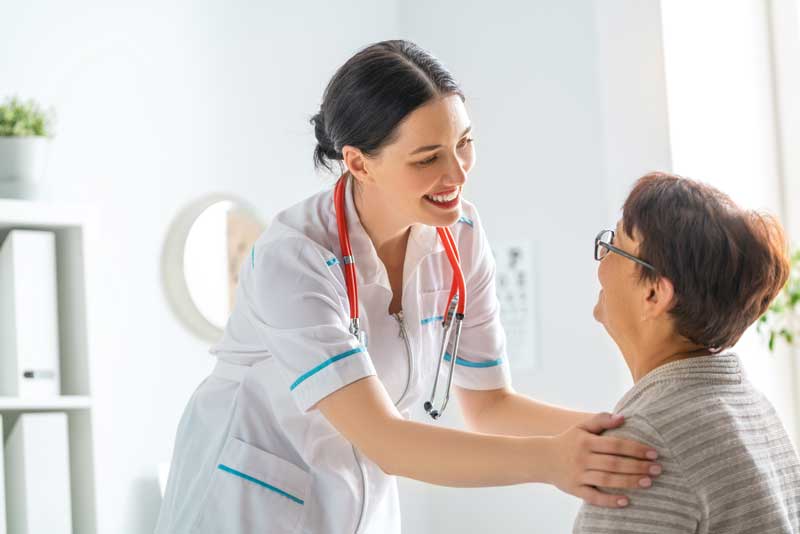
Patient engagement
Engaging with patients and establishing a consistent line of communication between them and their providers has proven to be a difficult challenge for healthcare systems across the country. Missed appointments and neglected treatment regimens can result in inadequate care as well as limit a healthcare systems’ ability to allocate resources effectively. That said, the situation isn’t hopeless.
With predictive analytics, healthcare systems can utilize a patient’s historical attendance data to forecast which patients pose a high risk for no-shows or poor adherence to prescribed treatment plans. These insights can then be used by providers to implement early interventions or establish a more frequent contact schedule with their patients.
This forecasting ability can also be applied in the care of patients diagnosed with chronic conditions. Using predictive risks scores derived from patient data, providers can design individualized treatment strategies that allow patients to independently track their treatment and engage with their own health. Hospitals need to be able to meet patients where they are, which includes finding alternatives to in-person care if a patient frequently misses appointments or struggles with current treatment regiments.
The same strategy is currently being used by behavioral health clinicians. Different mental illnesses and disabilities can all present themselves in wildly different ways. A patient prone to manic episodes and risky behavior requires a different approach than a bedridden patient suffering from depression. With this information, mental health providers can better coordinate their patient care, as well as ensure patients stay engaged and adhere to their treatment plans. This could be anything from simply modifying one’s schedule to hospitalizing the patient in question.
Outside of individualized treatment plans, a better understanding of patient behavior is all-around vital to developing physician-patient relationships. The stronger the relationship between a physician and their patient, the more likely they are to engage with their provider and adhere to regular screenings and follow-up appointments.
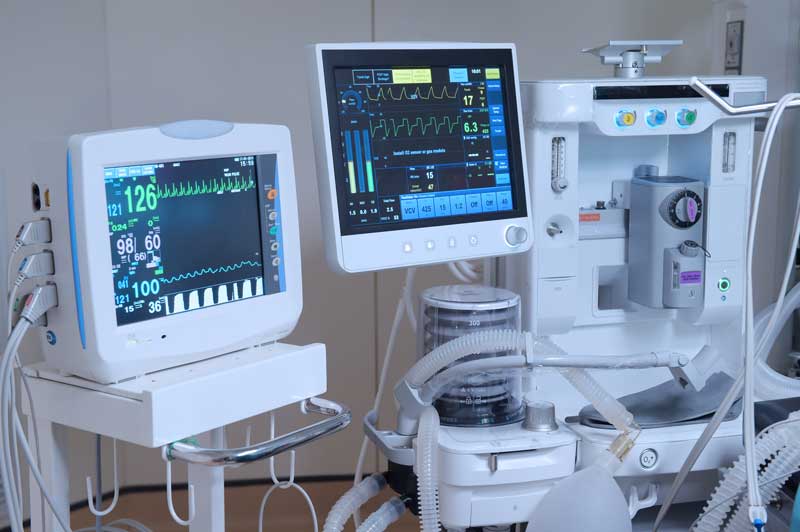
Resource allocation
COVID-19 highlighted how destructive large-scale disruptions can be for healthcare supply-chains, with hospitals suffering from massive shortages of ventilators, masks, gloves, and other PPE. Unfortunately, due to the raw scale of healthcare systems, even a statistically small margin of resource misallocations can have far-reaching consequences. In order to prepare for future disruptions, healthcare systems need visibility into how and where their resources are being utilized.
With data analytics, hospitals can anticipate and respond to potential shortages by identifying weak links in their supply chain—which inventory is falling behind and where. This visibility allows hospitals to optimize their resource management as well as allocate manpower to areas in need of the most support.
When shortages do occur, hospitals need to be able to base allocation policies on criteria such as urgency of need, likelihood and anticipated duration of successful treatment, and change in quality of life. However, none of this information is accessible without an analytics platform that can communicate real-time triage data between nurses and physicians.
The benefits of applying analytics in resource allocation also go beyond just large-scale disruptions. With predictive analytics, hospitals can identify seasonal or regional patterns in resource consumption, and in turn develop more agile resource management practices to prepare for future needs. When it comes to day-to-day operations, better resource management can also reduce wasteful spending.
Conclusion
Although healthcare systems have come a long way from conducting all their analysis on pen and paper, they still have a lot of room for improvement when it comes to maxing the value of their data. Revenue leaks, operational inefficiencies, and subpar patient outcomes remain major hindrances for hospitals across the planet.
Thankfully, continuing advances in healthcare technology and increasing data-advocacy present a bright future for hospitals willing to put in the work necessary to drive meaningful change.
Dimensional Insight®, an industry-leader for healthcare analytics, has led the charge in transforming digital health for the past 30 years. Our industry experience, combined with cutting-edge technology, has allowed us to provide our clients with unparalleled service.
At the core of our solution lies Diver Platform®, built upon an integrated set of analytical services that work together seamlessly to help you assemble and analyze the most relevant information. Other approaches that rely on multiple technologies lack the flexibility to meet the nuanced challenges of the healthcare industry. With Dimensional Insight, you gain real-time speed, seamless integration of data sources, the flexibility to view data the way you want to see it, and the governance that provides a single source of truth for your organization.
Additionally, Dimensional Insight knows healthcare. Our staff have spent decades solving customers’ most daunting analytical problems and fine-tuning the Diver Platform.
There’s a reason we have won Best in KLAS in healthcare analytics 8 times, more than any other vendor. It’s because we get the results our customers are looking for. Learn more about what our customers say to KLAS Research about us here:
https://www.dimins.com/awards/8-times-best-in-klas-winner/.
Interested in learning more?
References
- https://pubmed.ncbi.nlm.nih.gov/34402830/
- https://datacenter.commonwealthfund.org/topics/mortality-amenable-health-care
- https://www.ama-assn.org/practice-management/physician-health/medicine-s-great-resignation-1-5-doctors-plan-exit-2-years
- https://www.bls.gov/ooh/healthcare/nurse-anesthetists-nurse-midwives-and-nurse-practitioners.htm#tab-6
Download white paper:
Healthcare Analytics:
How to Tap into the Data that Will Drive Meaningful Change
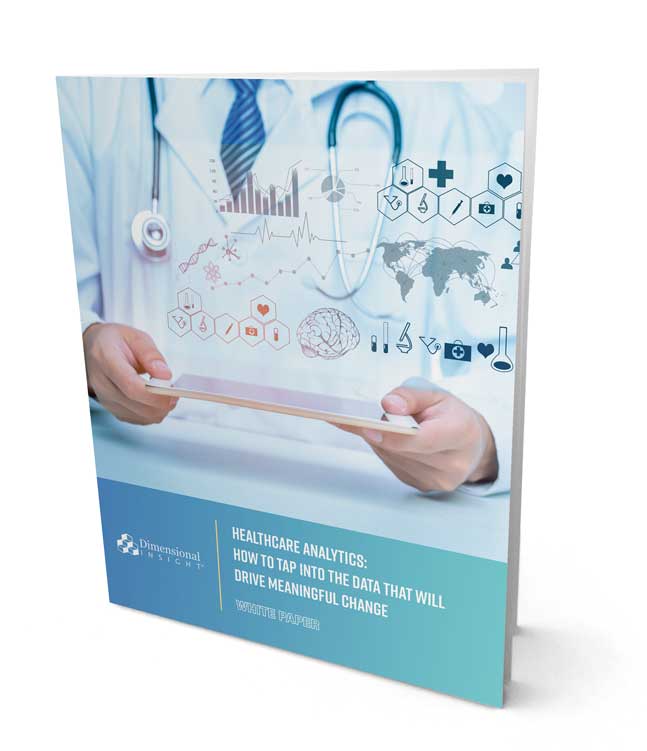
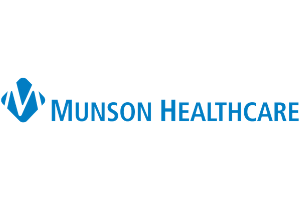
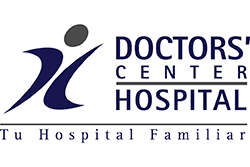

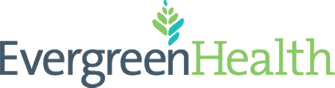
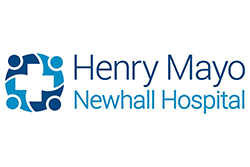